AI-Powered Analytics for Business Growth
Explore how AI-driven analytics tools are enabling businesses to gain actionable insights, forecast trends, and make data-driven decisions.
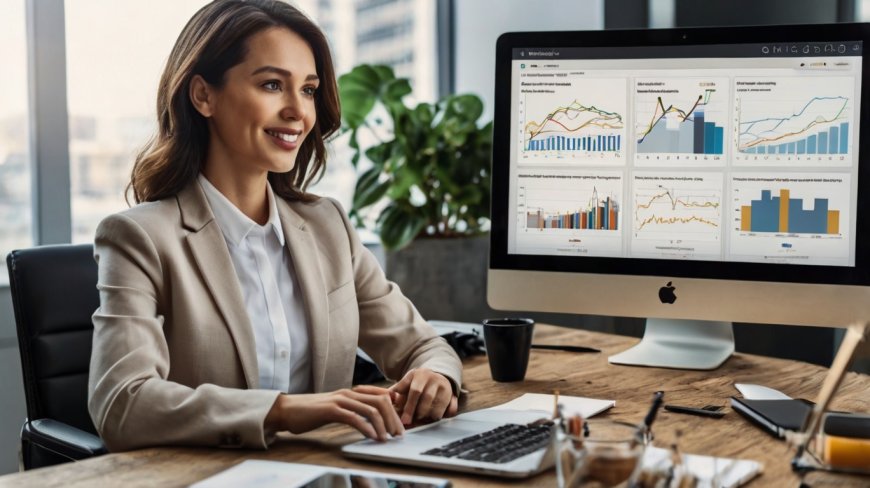
AI-Powered Analytics for Business Growth
In the era of data-driven decision-making, artificial intelligence (AI) has become a cornerstone for businesses seeking to achieve sustained growth. AI-powered analytics enable organizations to extract actionable insights from vast amounts of data, providing the tools to forecast trends, optimize operations, and make informed strategic decisions. By leveraging machine learning, natural language processing (NLP), and predictive analytics, businesses can gain a competitive edge in an increasingly dynamic market.
This blog explores how AI-powered analytics drive business growth, the benefits they offer, their challenges, and real-world applications.
What Are AI-Powered Analytics?
AI-powered analytics refer to the use of artificial intelligence techniques to analyze data, identify patterns, and deliver actionable insights. Unlike traditional analytics, which rely on manual processing and predefined models, AI-powered systems learn from data and adapt to changes, making them more accurate and efficient.
Key Features of AI-Powered Analytics:
-
Predictive Analytics: Uses historical data to predict future trends and behaviors.
-
Descriptive Analytics: Summarizes past data to understand what has happened.
-
Prescriptive Analytics: Suggests actions to achieve desired outcomes.
-
Real-Time Analysis: Processes and analyzes data as it is generated.
-
Automated Reporting: Generates insights and visualizations without manual intervention.
How AI-Powered Analytics Drive Business Growth
-
Improved Decision-Making
-
AI provides data-driven insights that help businesses make informed decisions, reducing the risks associated with guesswork.
-
Example: Retailers use AI to determine optimal pricing strategies based on demand forecasts and competitor analysis.
-
-
Enhanced Customer Experience
-
AI analyzes customer behavior to deliver personalized recommendations and improve engagement.
-
Example: Streaming platforms like Netflix leverage AI to recommend content tailored to individual preferences.
-
-
Operational Efficiency
-
AI optimizes processes, reduces inefficiencies, and automates routine tasks, allowing businesses to focus on strategic initiatives.
-
Example: Manufacturers use AI to predict equipment maintenance needs, minimizing downtime.
-
-
Market Trend Identification
-
AI detects emerging trends and provides insights into market dynamics, enabling businesses to stay ahead of competitors.
-
Example: E-commerce platforms analyze shopping trends to stock popular items and launch targeted campaigns.
-
-
Risk Management
-
AI identifies potential risks, such as fraud or supply chain disruptions, and provides solutions to mitigate them.
-
Example: Financial institutions use AI to detect fraudulent transactions in real time.
-
Benefits of AI-Powered Analytics
-
Scalability
-
AI systems can process vast amounts of data from multiple sources, making them suitable for businesses of all sizes.
-
-
Real-Time Insights
-
With AI, businesses can monitor and analyze data in real time, enabling quicker responses to changes.
-
-
Cost Savings
-
Automating data analysis reduces labor costs and improves operational efficiency.
-
-
Data Accuracy
-
Machine learning algorithms minimize human errors and enhance the reliability of insights.
-
-
Personalization
-
AI tailors products, services, and marketing efforts to meet individual customer needs.
-
Challenges of AI-Powered Analytics
-
Data Quality and Availability
-
Poor data quality or insufficient data can hinder AI’s effectiveness.
-
Solution: Implement robust data governance practices to ensure data integrity.
-
-
Complexity of Implementation
-
Integrating AI systems into existing workflows can be challenging.
-
Solution: Partner with experienced vendors or invest in skilled personnel.
-
-
Cost of AI Adoption
-
High initial investments may deter smaller businesses from adopting AI.
-
Solution: Start with scalable AI solutions and focus on high-impact use cases.
-
-
Ethical and Privacy Concerns
-
Misuse of data or biased algorithms can harm customer trust.
-
Solution: Ensure transparency, fairness, and compliance with data protection regulations.
-
-
Skill Gaps
-
Businesses may lack the expertise required to manage and optimize AI systems.
-
Solution: Provide training and upskilling opportunities for employees.
-
Real-World Applications of AI-Powered Analytics
-
Retail
-
Personalizing shopping experiences, optimizing inventory, and predicting demand.
-
Example: Amazon’s recommendation engine drives a significant portion of its sales.
-
-
Healthcare
-
Diagnosing diseases, predicting patient outcomes, and optimizing hospital operations.
-
Example: AI tools analyze medical imaging to detect early signs of cancer.
-
-
Finance
-
Fraud detection, credit scoring, and algorithmic trading.
-
Example: PayPal uses AI to monitor and prevent fraudulent transactions.
-
-
Marketing
-
Optimizing ad spend, targeting audiences, and analyzing campaign performance.
-
Example: AI-powered tools like HubSpot automate marketing workflows and provide actionable insights.
-
-
Supply Chain
-
Predicting demand, optimizing routes, and managing inventory.
-
Example: Logistics companies use AI to improve delivery efficiency and reduce costs.
-
Best Practices for Implementing AI-Powered Analytics
-
Define Clear Goals
-
Identify specific business problems that AI can address and set measurable objectives.
-
-
Invest in Quality Data
-
Prioritize data collection, cleaning, and storage to ensure AI systems work effectively.
-
-
Start Small
-
Begin with pilot projects to test AI capabilities before scaling up.
-
-
Focus on Collaboration
-
Encourage collaboration between data scientists, IT teams, and business units.
-
-
Monitor and Optimize
-
Continuously evaluate AI performance and make adjustments to improve outcomes.
-
Future Trends in AI-Powered Analytics
-
Integration with IoT
-
Combining AI and IoT to analyze real-time data from connected devices.
-
-
Explainable AI (XAI)
-
Developing AI systems that provide transparent and understandable insights.
-
-
Edge Analytics
-
Processing data at the edge of networks to reduce latency and enhance performance.
-
-
Automated Decision-Making
-
Using AI to automate complex decisions across various business functions.
-
-
AI-Driven Augmented Analytics
-
Leveraging AI to automate data preparation, insight generation, and visualization.
-
Conclusion
AI-powered analytics are revolutionizing how businesses leverage data for growth. By providing real-time insights, automating complex processes, and enabling personalized customer experiences, AI equips organizations to navigate a competitive landscape effectively. While challenges exist, businesses that strategically adopt AI analytics will unlock significant opportunities for innovation and success.
As AI technologies continue to evolve, their integration into analytics will further transform industries, making data-driven decision-making a standard practice in achieving long-term business growth.