Natural Language Processing (NLP): Powering the Next Generation of AI
Learn how NLP is enabling machines to understand and interact with human language across various applications.
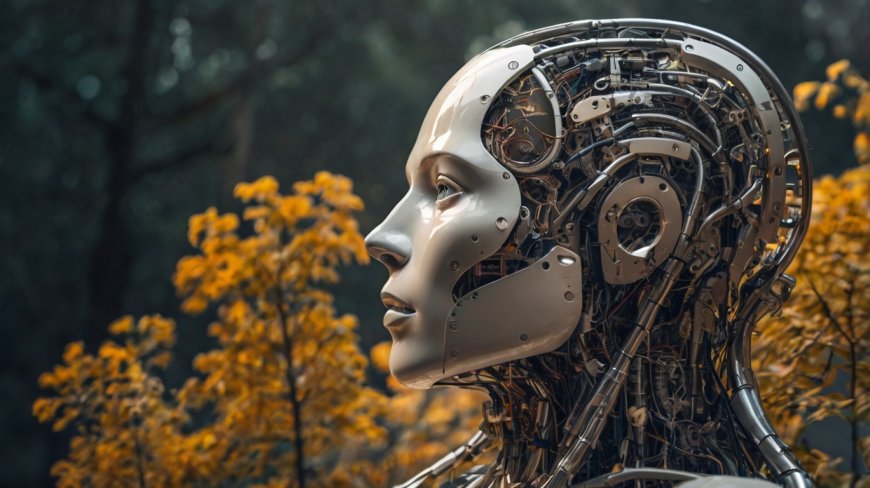
Natural Language Processing (NLP): Powering the Next Generation of AI
Natural Language Processing (NLP) is at the forefront of artificial intelligence (AI), enabling machines to understand, interpret, and respond to human language. As one of the most transformative AI disciplines, NLP powers technologies like chatbots, voice assistants, translation tools, and sentiment analysis. By bridging the gap between human communication and machine understanding, NLP is shaping the next generation of intelligent systems across industries.
This blog delves into the fundamentals of NLP, its applications, benefits, challenges, and future trends in advancing AI capabilities.
What is Natural Language Processing?
Natural Language Processing is a subfield of AI that focuses on enabling computers to process and analyze human language in text or speech form. It combines computational linguistics, machine learning, and deep learning to teach machines how to understand context, intent, and semantics.
Key Components of NLP:
-
Tokenization: Breaking text into individual words or phrases.
-
Part-of-Speech Tagging: Identifying the grammatical role of each word.
-
Named Entity Recognition (NER): Extracting specific entities like names, dates, and locations.
-
Sentiment Analysis: Determining the emotional tone of text.
-
Machine Translation: Translating text from one language to another.
-
Text Summarization: Generating concise summaries of lengthy texts.
Applications of NLP
-
Chatbots and Virtual Assistants
-
NLP enables chatbots and assistants to understand and respond to user queries naturally.
-
Example: Siri, Alexa, and Google Assistant use NLP to provide information, set reminders, and perform tasks.
-
-
Sentiment Analysis
-
Businesses use NLP to gauge customer sentiments from reviews, social media, and surveys.
-
Example: Companies analyze product reviews to improve customer satisfaction.
-
-
Machine Translation
-
NLP powers translation tools that convert text between languages.
-
Example: Google Translate uses NLP for real-time language translation.
-
-
Text Summarization
-
NLP algorithms extract key points from lengthy documents or articles.
-
Example: News aggregators use NLP to provide concise summaries of breaking news.
-
-
Healthcare
-
NLP extracts insights from medical records, streamlines documentation, and assists in diagnostics.
-
Example: IBM Watson Health leverages NLP to analyze clinical notes and suggest treatments.
-
-
Search Engines
-
NLP improves search algorithms to understand user intent and provide relevant results.
-
Example: Google’s BERT model enhances search accuracy by understanding context.
-
-
Customer Support
-
Automates responses and prioritizes tickets based on sentiment and urgency.
-
Example: Zendesk uses NLP to streamline customer support workflows.
-
-
Content Moderation
-
NLP detects and filters harmful or inappropriate content on platforms.
-
Example: Social media platforms use NLP to identify hate speech or spam.
-
Benefits of NLP
-
Improved User Experience
-
Enhances communication between humans and machines, making technology more accessible.
-
-
Automation of Repetitive Tasks
-
Reduces manual effort in tasks like data entry, content filtering, and document analysis.
-
-
Enhanced Decision-Making
-
Extracts actionable insights from unstructured data like customer feedback and social media posts.
-
-
Scalability
-
NLP solutions handle large volumes of data efficiently, supporting enterprise-level operations.
-
-
Cost Savings
-
Automating tasks with NLP reduces operational costs and improves efficiency.
-
Challenges in NLP
-
Ambiguity in Language
-
Words and sentences often have multiple meanings based on context.
-
Solution: Advanced context-aware models like transformers address linguistic ambiguity.
-
-
Language Diversity
-
Developing NLP models for less commonly spoken languages can be resource-intensive.
-
Solution: Use transfer learning to adapt models to multiple languages efficiently.
-
-
Bias in Data
-
NLP models trained on biased datasets may produce discriminatory results.
-
Solution: Ensure diverse and representative training datasets.
-
-
Data Privacy
-
Processing sensitive information raises privacy concerns.
-
Solution: Use encryption and adhere to data protection regulations.
-
-
Real-Time Processing
-
Delivering accurate responses quickly requires significant computational resources.
-
Solution: Optimize models for speed without sacrificing accuracy.
-
Key NLP Techniques and Models
-
Bag of Words (BoW):
-
Represents text as a collection of word frequencies.
-
-
TF-IDF (Term Frequency-Inverse Document Frequency):
-
Highlights important words in a document based on their frequency.
-
-
Word Embeddings:
-
Converts words into numerical vectors, capturing semantic relationships.
-
Example: Word2Vec, GloVe.
-
-
Transformers:
-
Advanced models that handle context and long-range dependencies in text.
-
Example: BERT, GPT-3.
-
-
Recurrent Neural Networks (RNNs):
-
Used for sequential data, such as text or speech.
-
Future Trends in NLP
-
Conversational AI
-
More sophisticated chatbots and voice assistants capable of complex interactions.
-
-
Multimodal NLP
-
Combining text, images, and audio for richer AI capabilities.
-
-
Zero-Shot Learning
-
Models that perform tasks without specific training, enabling faster deployment.
-
-
Low-Resource Language Processing
-
Increased focus on developing NLP models for underrepresented languages.
-
-
Explainable AI (XAI)
-
Enhancing transparency in NLP models to build trust and ensure ethical usage.
-
Real-World Example: ChatGPT
ChatGPT, powered by OpenAI’s GPT architecture, demonstrates the potential of NLP in understanding and generating human-like text. It has applications ranging from customer support to creative writing and education.
Capabilities:
-
Generates detailed responses based on context.
-
Handles follow-up questions and maintains conversational flow.
-
Assists in tasks like drafting emails, creating content, and providing technical support.
Conclusion
Natural Language Processing is driving the next generation of AI by enabling machines to understand and interact with human language effectively. From enhancing customer experiences to automating workflows, NLP is revolutionizing industries and unlocking new possibilities for innovation.
As advancements in NLP continue, addressing challenges like bias, privacy, and resource limitations will be essential. By prioritizing ethical practices and inclusivity, NLP can shape a future where AI-powered systems enhance human communication and decision-making on an unprecedented scale.