Low-Code AI: Simplifying Artificial Intelligence Implementation
Learn how low-code platforms are democratizing AI, making it accessible to businesses without technical expertise.
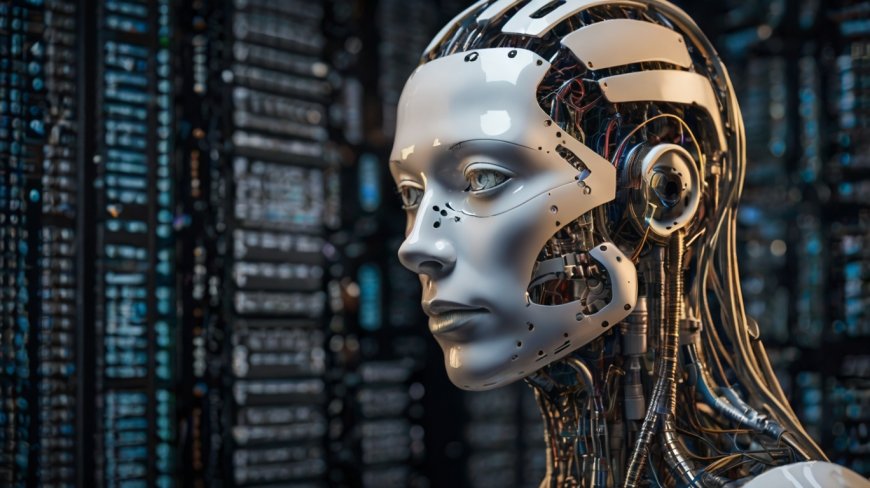
Low-Code AI: Simplifying Artificial Intelligence Implementation
Artificial Intelligence (AI) is transforming industries by enabling smarter decision-making, automating processes, and uncovering insights from data. However, implementing AI traditionally requires technical expertise, significant resources, and time—barriers that many organizations struggle to overcome. Low-code AI solutions are changing the game by democratizing AI development, making it accessible to businesses of all sizes.
This blog explores the concept of low-code AI, its benefits, use cases, challenges, and the role it plays in accelerating AI adoption across industries.
What is Low-Code AI?
Low-code AI refers to platforms and tools that enable users to develop, deploy, and manage AI models with minimal coding. These platforms provide drag-and-drop interfaces, pre-built templates, and automated workflows, allowing non-technical users to create AI solutions quickly and efficiently.
Key Features of Low-Code AI Platforms:
- Visual Development: Drag-and-drop tools for building AI workflows.
- Pre-Built Models: Ready-to-use algorithms for common AI tasks.
- Integration Tools: APIs and connectors for integrating with existing systems.
- Automated Machine Learning (AutoML): Simplifies the process of training, testing, and optimizing AI models.
- Scalability: Cloud-based deployment options for scaling AI solutions.
Benefits of Low-Code AI
-
Accelerated Development
- Reduces the time required to develop AI applications by automating complex tasks.
- Example: Creating a sentiment analysis model in hours instead of weeks.
-
Accessibility for Non-Experts
- Enables business analysts, marketers, and other non-technical users to create AI solutions without extensive coding knowledge.
-
Cost Efficiency
- Reduces the need for large AI development teams and expensive infrastructure.
-
Faster Prototyping
- Allows organizations to quickly test and iterate on AI solutions, reducing the risk of investing in ineffective models.
-
Improved Collaboration
- Facilitates collaboration between technical and non-technical teams, aligning AI projects with business goals.
-
Seamless Integration
- Simplifies the integration of AI solutions with existing tools and workflows, maximizing ROI.
Use Cases for Low-Code AI
-
Customer Support Automation
- Build chatbots and virtual assistants to handle customer queries, reducing response times.
- Example: A retailer uses low-code AI to create a chatbot for tracking orders and answering FAQs.
-
Predictive Analytics
- Develop models to forecast sales, customer churn, or inventory needs.
- Example: A subscription service predicts customer churn using a low-code AI platform.
-
Fraud Detection
- Identify unusual patterns in transactions to prevent fraud.
- Example: A fintech company uses low-code AI to flag suspicious credit card transactions.
-
Marketing Personalization
- Create personalized recommendations and campaigns based on user behavior.
- Example: An e-commerce platform uses low-code AI to recommend products to customers.
-
Employee Productivity Tools
- Automate repetitive tasks like data entry, document classification, or email sorting.
- Example: A law firm automates contract analysis using low-code AI.
-
Image and Video Analysis
- Process visual data for tasks like quality inspection, facial recognition, or object detection.
- Example: A manufacturing company uses low-code AI to identify defects in product images.
Challenges of Low-Code AI
-
Limited Customization
- Pre-built templates may not address highly specific business needs.
- Solution: Opt for platforms that allow custom coding for advanced use cases.
-
Data Quality Dependency
- The accuracy of AI models relies on high-quality, well-prepared data.
- Solution: Invest in data cleaning and preprocessing before model development.
-
Scalability Concerns
- Some low-code platforms may struggle with scaling complex AI solutions.
- Solution: Choose platforms with proven scalability for enterprise use.
-
Security and Compliance
- Handling sensitive data with third-party tools raises privacy concerns.
- Solution: Ensure platforms comply with regulations like GDPR or HIPAA.
-
Skill Gap
- While low-code AI reduces technical barriers, some understanding of AI concepts is still necessary.
- Solution: Provide training and resources for users to maximize platform potential.
Popular Low-Code AI Platforms
-
Google AutoML
- Simplifies the creation of custom machine learning models with minimal coding.
-
Microsoft Power Platform
- Offers AI Builder for creating models integrated with business applications.
-
DataRobot
- Provides automated machine learning tools for building and deploying AI solutions.
-
H2O.ai
- Focuses on open-source AI tools with easy-to-use interfaces.
-
AWS SageMaker Canvas
- A no-code tool for building machine learning models on Amazon Web Services.
Future Trends in Low-Code AI
-
Increased Adoption Across Industries
- More industries, including healthcare, finance, and retail, will adopt low-code AI for diverse applications.
-
Advanced AutoML Capabilities
- Platforms will offer more sophisticated AutoML features for fine-tuning complex models.
-
AI-Powered Low-Code Development
- AI will assist in automating even more aspects of the development process, from data preparation to model deployment.
-
Focus on Ethical AI
- Low-code platforms will integrate features to ensure transparency, fairness, and compliance in AI models.
-
Integration with IoT and Edge Computing
- Low-code AI will expand to support real-time analytics for IoT devices and edge computing scenarios.
Best Practices for Using Low-Code AI
-
Define Clear Objectives
- Identify specific problems you want to solve with AI and align them with business goals.
-
Prepare High-Quality Data
- Ensure data is clean, relevant, and representative to improve model accuracy.
-
Start Small
- Begin with simple use cases to build confidence and gradually scale up.
-
Monitor and Refine Models
- Continuously evaluate model performance and retrain as needed to maintain accuracy.
-
Invest in User Training
- Provide resources to help non-technical users understand the platform’s capabilities and limitations.
Conclusion
Low-code AI is revolutionizing the way businesses adopt artificial intelligence, breaking down barriers to entry and empowering teams to innovate faster. By simplifying the development process, these platforms enable organizations to harness AI’s potential without extensive technical expertise or resources.
As low-code AI continues to evolve, it will play a pivotal role in democratizing AI, fostering innovation, and driving business growth. Organizations that embrace this technology will be well-positioned to stay competitive in an increasingly data-driven world.